A research team of Seoul St. Mary’s Hospital has developed a model that predicts rectal cancer using patients' radiological, pathological, and clinical data.
The selected cohort model of 489 patients had rectal cancer surgery from 2009 to 2015. To develop the predictive model for recurrence in rectal cancer, researchers collected radiologic, and clinicopathologic data made a multifaceted regression analysis.
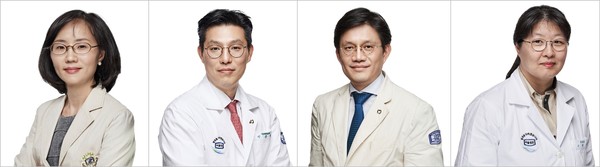
Of the 20 risk factors of cancer recurrence, they found four factors that show the worst prognosis – nodal metastasis, perineural invasion, MR-based external venous invasion, and cancer found on a short distance from the anal verge (within 5cm). These risk factors each showed 2.59, 2.29, 2.09, and 1.69 times higher recurrence risk chances than the control group.
Professors Oh Soon-nam of the Department of Radiology, Lee Yoon-suk and Lee In-kyu of the Department of Colorectal Surgery, and Lee Myung-ah of the Department of Oncology led the study.
They divided the patients into three cohort groups using a prediction model based on these risk factors – low, medium, and high-risk groups of recurrence. They found their chances of recurrence were 13.4 percent, 35.3 percent, and 61.5 percent, respectively.
The team proved the new model showed statistically superior predictability compared to that of conventional models.
Because colorectal cancer has only 40-50 percent of five-year survival rate after the surgery, follow-up tests are as important as the surgery. Therefore, the team announced that they would make a follow-up protocol personalized for high-risk patients based on the cancer recurrence predictive model.
“Using the big data, we plan to construct personalized tracking test protocol with a variety of AI algorithms,” Professor Oh said. “We are pursuing personalized follow-up test measures that perform a detailed early diagnosis of risk factors and reduces unnecessary examination for low-risk patients.”
The team is also researching to develop AI-based diagnosis, the professor added.
The research result was published in the online edition of European Radiology on May 4.