Researchers at the Korea Institute of Science and Technology (KIST) have developed an artificial intelligence technology that generates computed tomography based on magnetic resonance imaging images.
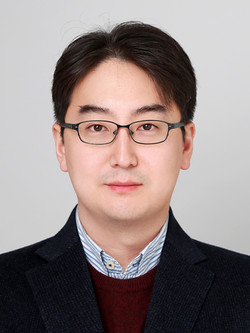
The team, led by Doctor Kim Hyung-min of the Bionics Research Center at KIST, hopes that the technology will greatly help hospitals perform transcranial focused ultrasounds with MRI alone.
According to the team, transcranial focused ultrasound is a technology that can treat degenerative movement disorders, intractable pain, and mental disorders by delivering ultrasound energy to a specific area of the brain without opening the skull.
"In using this method, doctors must use the treatment with image-based technology to exactly locate the brain lesion," the team said. "Therefore, information about the patient's skull, which is difficult to identify with MRI alone, doctors conventionally used CT along with MRI to accurately focus the ultrasound through the skull on the brain lesion. However, there were concerns about the safety of CT scans, which require frequent radiation exposure.”
As a solution, the team noted that there had been efforts to obtain skull information from MRI images. However, such a process required special MRI coil equipment or imaging technology not widely used in the medical field. To resolve this problem, Doctor Kim's team proved that it is possible to perform a transcranial focused ultrasound with MRI alone by using this technology.
In developing the AI platform, the team used a 3D conditional adversarial generative network that learns the nonlinear CT transformation process from T1-weighted MRI images, one of the most used images in the medical field.
Afterward, they devised a loss function that minimized the Hounsfield unit pixel variation error of CT images and optimized the neural network performance by comparing synthetic CTs' quality changes according to the normalization methods of MRI image signals.
"By using our AI system, patients can receive focused ultrasound treatment without worrying about radiation exposure, and we expect that the technology will also reduce that time and economic costs by simplifying the medical staff's work process by omitting the process of taking and aligning additional images," Doctor Kim said.
By conducting follow-up researches to understand the error rate according to ultrasound parameters and transducers and the possibility of applying AI CT to various parts of the body, the team plans to expand the platform's application to various treatment technologies, Kim added.
The research results were published in the latest issue of IEEE Journal of Biomedical and Health informatics.