A team of researchers at the Korean Advanced Institute of Science (KAIST) has successfully developed a brain-machine interface (BMI) that decodes human brain signals to control robot arms without the need for long-term training.
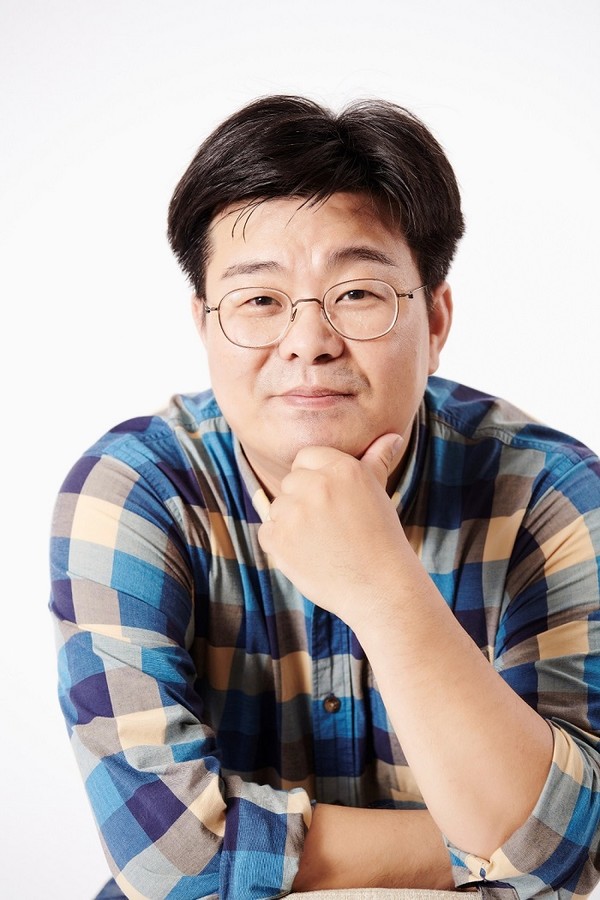
BMI is drawing attention as an auxiliary technology that allows patients with disabilities or amputations to control robot arms to recover arm movements necessary for daily life.
Decoding technology is used to measure electrical signals generated from the brain when humans move their arms and decode brain signals through artificial intelligence (AI) analysis via machine learning.
However, the difficulty of predicting the exact direction of the arm has long been a challenge because the imaginary brain signal has a significantly lower signal-to-noise ratio than the actual arm movement. Previous studies tried using other body movements with higher signal-to-noise ratios to move the artificial arm, but the difference between the intended arm extension and cognitive movements often causes inconveniences for the user.
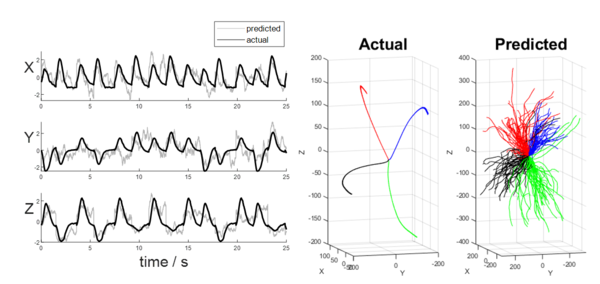
Consequently, the research team developed a variable Bayesian least square machine learning technique to measure the user's natural arm motion imagination using an electrocorticogram with excellent spatial resolution. They also calculated the direction information of arm movements that are difficult to measure directly.
According to the researchers, this technology is not limited to specific cerebral areas so it can output optimal computational model parameter results by customizing imaginary signals and cerebral area characteristics that may vary from user to user.
The researchers confirmed that the patient's imagined arm extension direction can be predicted with up to 80 percent accuracy through cerebral cortical signal decoding.
Furthermore, by analyzing the computational model, they confirmed that the closer the cognitive process of imagining is to the actual extension of the arm, the higher the accuracy of the predicted arm direction.
Professor Jeong Jae-seung who led the study said, "This customized brain signal technology which can control robot arms without long-term training is expected to greatly contribute to commercializing robot arms to replace prosthetic arms for disabled persons in the future."
First author Jang Sang-jin, added, "We were able to accurately predict the trajectory of the arm through cerebral cortical analysis of imaginary signals alone, so users can now control the device only with their thoughts."
The study, “Decoding projects of imaged hand movement using electronic graphs for brain-machine interface, was published in the Journal of Neural Engineering.