KAIST researchers have developed and verified a deep learning algorithm, that can detect differences in gene expression patterns between tumor cells and normal cells based on logic circuits, to optimize chimeric antigen receptor (CAR) cell therapies.
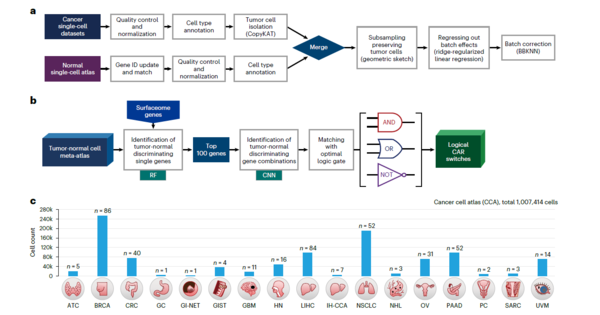
Identifying optimal target antigens that distinguish cancer cells from normal surrounding tissue cells remains a key challenge in (CAR) cell therapy for tumors with intratumoral heterogeneity. Accordingly, the team led by Professor Choi Jung-kyoon of the Bio and Brain Engineering Department at KAIST developed a method to accurately target cancer cells without side effects by successfully differentiating cancer and normal cells.
There are several ways to overcome cancer by utilizing the immune system of cancer patients, including immunosuppressants and cancer vaccines, as well as cell therapy. In particular, immune cells called CAR-T or CAR-NK equipped with chimeric antigen receptors can recognize cancer antigens and directly destroy cancer cells.
Despite successes in CAR cell therapy in blood cancers, developing CAR cells that have effective cancer-killing ability while minimizing side effects for solid cancers have been difficult.
In recent years, the development of smart immune cells that can effectively target cancer cells by utilizing computational logic circuits such as AND, OR, and NOT, has been increasing.
In this regard, the researchers established a large-scale cancer and normal single-cell database to discover genes that are expressed only in cancer cells at the cell level. The researchers then developed an artificial intelligence algorithm that selects gene pairs that can best distinguish cancer cells from normal cells. In particular, this algorithm was used to find logic circuits that can specifically target cancer cells through cell-level simulations of all gene combinations.
Accordingly, single-cell transcriptome-coupled epitope profiling validated the AND, OR, and NOT switch targets in ovarian cancer and colorectal cancer. Consequently, CAR immune cells equipped with logic circuits are expected to minimize side effects while maximizing the effectiveness of chemotherapy.
"This study presented a methodology that has never been attempted before and succeeded in finding optimal CAR cell circuits through simulation of millions of individual cancer cells and normal cells," Dr. Kwon Joon-ha of KAIST’s Bio and Brain Engineering Department said. “As a breakthrough technology that applies artificial intelligence and computational logic circuits to immune cell engineering, we expect this research to play a key role in expanding CAR cell therapies to solid tumors."
The study was published in the latest issue of Nature Biotechnology on Feb. 16.
Related articles
- KAIST develops customized drug discovery platform
- KAIST researchers identify potential method to treat intractable epilepsy in kids
- Reprogramming lung cancer cells can revert metastasis: KAIST
- SMC, KAIST find new cancer immunotherapy pathway
- KAIST develops cancer cell-specific targeting clathrin drug delivery system