NGeneBio said on Monday a study found that gene-specific machine-learning models are more efficient at predicting the pathogenicity of rare genetic variants than disease-specific machine-learning models.
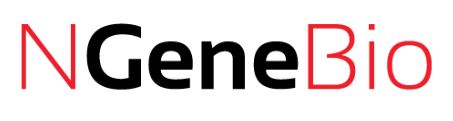
The research paper is about interpreting the pathogenicity of BRCA1/2 genetic variants, in rare diseases and hereditary cancers. Currently, the pathogenicity of genetic variants is determined based on publicly recorded databases, which contain information on only a small fraction of the most common variants.
Accordingly, a team of researchers led by Professor Hwang Kyu-baek of Computer Science and Engineering at Soongsil University devised a method to develop gene-specific models of rare variants that were difficult to interpret due to a lack of sufficient genomic information.
By applying various machine-learning models to 1,068 rare genetic variants in 28 genes associated with hereditary cancer, they were able to optimize a gene-specific machine-learning model with high accuracy.
While it is difficult to interpret how rare genetic variants are related to breast cancer due to small datasets, this study demonstrates that machine learning can effectively interpret rare genetic variants even with small datasets, said the researchers.
In particular, by applying the model to the prediction of pathogenic variants in the BRCA1/2 genes associated with breast cancer, the researchers were able to improve the accuracy of prediction and analysis compared to previous approaches.
Consequently, NGeneBio plans to apply the results of this study to its BRCAaccuTest PLUS test to improve the accuracy of the test result for patients with breast cancer who have previously been difficult to diagnose.
BRCAaccuTest PLUS is the first NGS-based precision diagnostic test for breast and ovarian cancer approved as a medical device by the Ministry of Food and Drug Safety in Korea which is utilized in domestic hospitals as well as overseas medical institutions in Singapore.
"By utilizing gene-specific machine learning to identify variants whose interpretation is not accurate and clearly distinguish between variants with medical value and variants that can be ignored, the accuracy of NGS-based precision diagnosis results has been improved," "Based on the results, we will expand the machine learning model to all NGS-based gene panel analyses to accurately diagnose disease gene variants that are difficult to diagnose."
"By utilizing gene-specific machine learning to identify variants with inaccurate interpretation and clearly distinguish between variants with medical value, we have improved the accuracy of NGS-based precision diagnosis results," said Kim Kwang-jung, head of NGeneBio’s research center. "Based on the results, we will expand our model to all NGS-based gene analyses to accurately discern gene variants that are difficult to diagnose."
The results were published in the international journal, Nature on June 28.
Related articles
- NGeneBio acquires tech to diagnose Alzheimer’s using blood
- NGeneBio, Agilent Technologies Korea to expand NGS business
- Amorepacific jumps into DTC gene test market
- NCC, Korea University license out prognostic breast cancer biomarker to CbsBiosciences
- Novomics expands gastric cancer patent to rectal cancer prognosis
- NGeneBio sets up AI subsidiary to tap into U.S. precision diagnosis market
- NGeneBio releases upgraded NGS-based companion diagnostics for cancer
- NGeneBio's blood cancer diagnostic panel gets permit in Thailand
- NGeneBio registers patent for NGS-based TB diagnosing tech