A team of researchers at Yonsei University College of Medicine (YUCM) succeeded in developing a deep learning-based model to diagnose autism spectrum disorder (ASD) and determine its severity.
ASD is a neurodevelopmental disorder characterized by abnormal patterns of social interaction and communication.
"Joint attention" is a behavioral pattern that occurs during interactions with others and consists of eye shifting, head-turning, and eye contact. Abnormal joint attention behavior in children is a key behavioral marker for the clinical diagnosis of ASD.
However, there is no objective, quantitative way to measure joint attention, so clinical assessors have to rely on their judgment to determine the presence and severity of autism.
The three main types of joint attention include initiation of joint attention (IJA) which occurs when the child directs the attention of another person towards a shared target. Meanwhile, low-level response to joint attention (RJAlow) and high-level response to joint attention (RJAhigh) describes when children look at objects in response to another person's initiation of an interaction, by pointing to a object at a close or distant location.
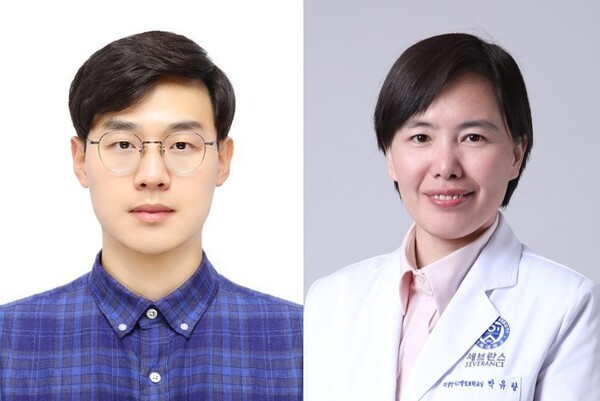
The researchers led by Lecturer Ko Chan-young and Professor Park Yu-rang of the Department of Biomedical Systems Informatics at YUCM developed a protocol to objectively assess joint attention. Using this protocol, they recorded videos of the three types of joint attention behaviors from 95 children, 45 with ASD, and 50 without ASD symptoms in children, aged 24-72 months.
Subsequently, the researchers trained a deep learning model using this sata to distinguish children with ASD and those without this disorder to predict the severity of ASD.
Consequently, the research team analyzed the three types of joint attention using the area under the receiver operating characteristic curve (AUROC), accuracy, precision and recall.
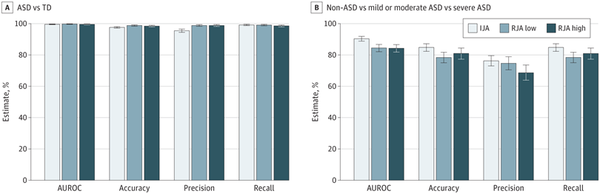
The AUROC was used to describe the diagnostic accuracy of a particular test for diagnosing a condition. Accuracy was used to describe the percentage of cases in which the predicted result and the actual value are the same out of the total dataset.
Precision was used to determine the probability that a person with a positive test result actually has a disease and recall determined the probability that a person with a real disease will test positive.
The predictive model had an AUROC of 99.6 percent, accuracy of 97.6 percent, precision of 95.5 percent, and recall of 99.2 percent when training on videos of IJA, in which the child guided the interaction. The model performed equally well when analyzing two other types of interactions as input.
For the severity assessment, which categorized the severity of ASD into no symptoms, mild-moderate, and severe, the AUROC was 90.3 percent, accuracy 84.8 percent, precision 76.2 percent, and recall 84.8 percent when IJA videos were analyzed as input, compared to the other types.
This suggests that IJA is a more precise classification that reflects children's desire and intentionality for social interaction than other types of collaboration.
"We developed a model that digitizes children's behavioral indicators to identify ASD and assess the severity of symptoms in an objective way," said Professor Park. "This will help develop screening and precision diagnostic tools for research and clinical evaluation of not only ASD but other neurological diseases with multiple behavioral problems."
The findings were published in the international journal JAMA Network Open.