A research team Korea Advanced Institute of Science and Technology (KAIST) has leveraged artificial intelligence (AI) to find a potential cause and treatment for schizophrenia.
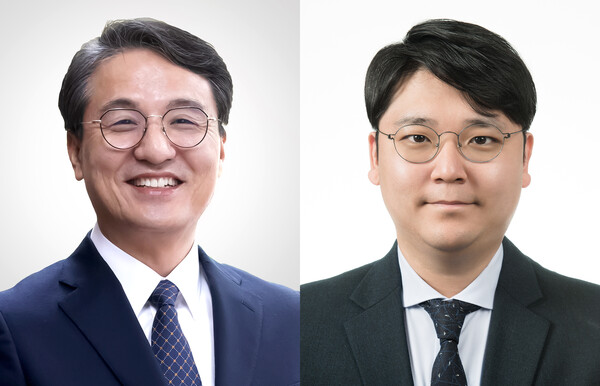
Schizophrenia is a mental disorder characterized by hallucinations, delusions, and cognitive disabilities. Schizophrenia has become a pressing societal issue in Korea, with notable incidents involving schizophrenic individuals, such as the knife wielding of a schizophrenic in Daejeon in August this year.
Despite the grave implications, the underlying causes of schizophrenia remain elusive and patients mainly rely on antipsychotic drugs like risperidone and clozapine for symptomatic relief.
The team, led by Professor Lee Do-heon of the Department of Bio and Brain Engineering, used AI to identify a congenital pathological model and markers predicting schizophrenia based on individual genotypes. The team cooperated with researchers from the Korea Institute of Oriental Medicine and the U.S.'s Stanley Medical Research Institute (SMRI).
Professor Lee's team adopted a cutting-edge AI approach known as explainable deep learning to the multi-level brain tissue data provided by SMRI.
This resulted in an artificial neural network model that elucidated the pathology between congenital genotypes and schizophrenia.
Interpreting the model, the team found that inborn genotype determines schizophrenia susceptibility by altering the development of prefrontal cortex and orbitofrontal cortex neurons in the brain through the regulation of gene and protein expression.
The team also proposed a genotype combination that reduces the density of neurons in the brain as a predictive marker for schizophrenia, opening up the possibility of personalized schizophrenia prediction and treatment of the cause of schizophrenia through cell therapy.
Emphasizing the need for "interpretable AI" over "black-box AI" in biomedical research, Professor Lee stated, "Compared to conventional AI, our study positioned nodes with specific biological meanings, such as gene names and cellular states, within the artificial neural network."
The team then analyzed their interrelationships using machine learning techniques, Lee added.
The research results were published in the September issue of Briefings in Functional Genomics.