Korean researchers developed a model to predict the probability of recurrence following double jaw surgery.
Double jaw is a condition in which the lower jaw is relatively less developed in comparison to the upper jaw, leading to a situation where the lower teeth are positioned more forward than the upper teeth. This not only results in issues with chewing and speech articulation but also affects the overall facial aesthetics.
To address this concern, bimaxillary surgery, which aligns the alignment of both the upper and lower jaws, can rectify this malocclusion.
However, over time, the jaw tends to revert to its original position, posing challenges for both surgeons and patients alike.
The research team led by Professor Kim Young-ho from the Department of Orthodontics at the Institute of Oral Health Science, Ajou University School of Medicine, and Professor Chae Hwa-sung from the Department of Orthodontics at Gwangmyeong Hospital, Chungang University, conducted a study in which they measured 55 linear and angular skeletal and dental surgical movements in 227 patients who underwent bimaxillary surgery. These measurements were taken at four distinct time points: before the commencement of treatment, before the surgical procedure, immediately after surgery, and after the end of treatment.
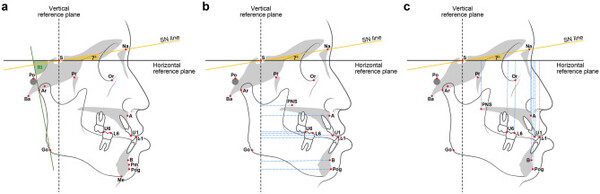
The research team employed machine learning techniques to construct a predictive model utilizing these measurements. When this model was applied to clinical data, it demonstrated an impressive 90 percent accuracy in forecasting the probability of the jaw relapsing forward.
Machine learning is one of the methods that empowers artificial intelligence (AI), a technology enabling computers to autonomously analyze substantial datasets and discern patterns for informed decision-making and future predictions.
The researchers noted that this is the first publication of a model utilizing machine learning for the prediction of the likelihood of recurrence following double jaw surgery.
The study's significance lies in its revelation that the direction of the jawbone's movement holds greater importance than the extent of the surgical procedure. By forecasting the risk of recurrence based on the degree of rotational movement in the lower jaw, the research team confirmed that a tailored surgical plan for each patient can substantially diminish the likelihood of relapse.
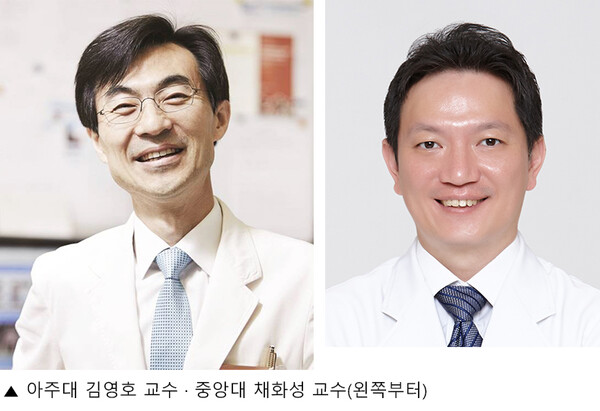
“Many people grappling with severe malocclusion express interest in bimaxillary surgery, yet they may be deterred by the associated high costs, risks of facial paralysis and excessive bleeding, as well as the potential for recurrence," Professor Chae said. "The ability to anticipate the risk of recurrence in advance could prove highly valuable in crafting a suitable treatment strategy."
Professor Kim noted that severe malocclusion can lead to daily discomfort, particularly during activities like eating and pronouncing words such as "smile" and "sport," which may sound like "tsmile" and "tsport." He also emphasized that orthognathic surgery can be conducted in adulthood once the growth phase is complete.
The study was published in October in Nature's sister journal, Scientific Reports, under the title, "The prediction of sagittal chin point relapse following two-jaw surgery using machine learning.”