Severance Hospital has developed an artificial intelligence (AI) model capable of rapidly and accurately diagnosing sepsis and predicting patient outcomes.
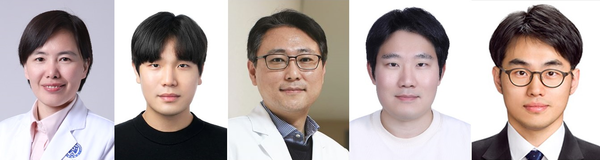
Sepsis, a critical condition resulting from an abnormal body response to infection leading to major organ dysfunction, has high incidence and mortality rates. Rapid early diagnosis and immediate intervention are crucial due to their complex and patient-specific immune responses and potential to quickly affect multiple organs, increasing the risk of death if treatment is delayed.
Current diagnostic biomarkers for sepsis, such as C-reactive protein (CRP) and procalcitonin (PCT), often show delayed responses, leading to late diagnoses. Other biomarkers like interleukin-6 (IL-6) lack standardization, complicating the interpretation of diagnostic results. These challenges underline the need for new biomarkers.
To provide a new biomarker, the team, led by Professor Park Yu-rang and researcher Kim Jong-hyun of the Department of Biomedical Systems Informatics at Yonsei University College of Medicine, Professor Chung Kyung-soo and instructor Sung Min-dong of the Department of Internal Medicine at Severance Hospital, and Min Hyun-seok of Tomocube, developed an AI model using 3D images of CD8 T cells.
The model boasted an impressive accuracy rate exceeding 99 percent.
In detail, the research team utilized CD8 T cell image data and AI models to explore the possibility of diagnosing and predicting the prognosis of sepsis.
Blood samples from eight sepsis recovery patients were used to isolate and photograph CD8 T cells at three different stages -- at the time of sepsis shock diagnosis (T1), resolution of sepsis shock (T2), and before discharge (T3).
The imaging was done using holotomography microscopy, a technique that allows rapid and stable acquisition of 3D images of living immune cells without the need for staining that could alter cell structure.
Afterward, the team captured the images at each stage and analyzed and compared them with those of a healthy control group of 20 individuals using a deep learning-based AI classification model.
Images taken at the time of sepsis shock diagnosis were used to assess the possibility of diagnosing sepsis shock, while images from surviving and non-surviving patient groups were utilized to predict the prognosis of sepsis shock.
The AI model's performance was evaluated using the receiver operating characteristic (ROC) area under the curve (AUROC), a statistical method indicating the diagnostic accuracy of a specific test tool for a particular disease.
An AUROC close to one indicates high performance, with scores above 0.8 considered high-performing models.
The analysis showed that by using a single-cell image, the AI model's predictive accuracy (AUROC) was 0.96 (96 percent), while using two-cell images increased the performance to over 0.99 (99 percent). Similarly, the prognosis prediction model showed an accuracy of 0.98 (98 percent) with a single cell image and over 0.99 (99 percent) with two.
"This research has elucidated the role of three-dimensional images of CD8 T cells as biomarkers for sepsis," Professor Chung said. "We expect the AI model to assist in making prompt and accurate diagnoses and prognosis predictions for sepsis patients, thereby aiding in personalized treatment decisions."
Related articles
- Moderate exercise enables longer lives for older adults despite fine dust: study
- YUHS unveils innovative DTx integration platform, revolutionizing personalized care
- Korean researchers find tear biomarker for early Alzheimer's detection
- Severance Hospital marks 'world's 1st' to perform 40,000 robotic surgeries
- Severance Hospital finds new causative factor for retinoblastoma
- Severance Hospital conducts 1st successful electroporation therapy for liver cancer
- 3-year-old heart transplant survivor discharged from Severance Hospital
- Plant-based hemostatic powder shows promise in treating gastrointestinal bleeding