Korean researchers have developed an algorithm to identify wakefulness and sleep stages using electroencephalographic (EEG) signals.
The researchers, led by Professors Hwang Hee and Kim Hun-min at Seoul National University Bundang Hospital (SNUBH)’s Pediatrics Department as well as Professor Yoon Sung-roh of Seoul National University (SNU)’s Electrical and Computer Engineering Department, published their study in the July issue of IEEE Acess.
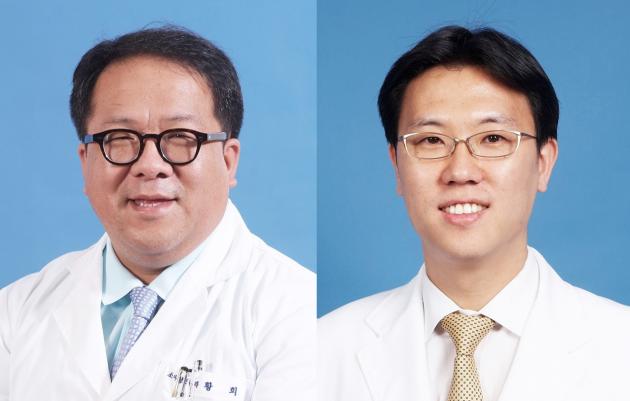
EEG signals are the only physiological marker in the central nervous system that can be measured in-vitro. EEG signals in sleep tests are essential for measuring adverse reactions in various neurological diseases.
To read EEG and polysomnography, the classification of consciousness is critical. Through EEG analysis, the consciousness is divided into wakefulness, REM sleep, and non-REM sleep.
However, these tests have been an enormous burden on both patients and physicians because they are time-consuming and labor-intensive because of the complicated process of applying various conditions at the same time and reading.
In the study, the researchers used a “hybrid neural network,” combining the convolutional neural network (CNN) with long-short term memory (LSTM), which is an advanced version from the EGG analysis model using machine-learning.
The research team of SNUBH analyzed EEG recordings of 218 children, and three skilled neurologists distinguished sleep stages up to 35,000 EEG units. Based on the data, SNU’s Artificial Intelligence Research Center developed an algorithm that automatically analyzes wakefulness and sleep stages.
The results showed that the accuracy of the algorithm was 96 percent in wakefulness and 92 percent in second-stage non-REM sleep.
“It is possible to minimize human error if we apply AI to EEG analysis, which requires years of training and expertise. As AI advances, we can conduct a more accurate and detailed EEG analysis, raise EEG analysis efficiency and quality,” Prof. Hwang said.
The study is part of machine-learning medical software “Dr. Answer” project, sponsored by the Ministry of Science and ICT and operated by the National IT Industry Promotion Agency (NIPA). The AI algorithm is expected to be installed in the module for distinguishing wakefulness in automatic EEG analysis software, under the epilepsy treatment task of the project.