Lunit has become Korea’s only AI-based medical image analytics company to present its research at the American Society of Clinical Oncology for two consecutive years.
The company recently made two online poster presentations during the American Society of Clinical Oncology (ASCO) 2020, proving the association between three biomarker immune phenotype – inflamed, excluded, and desert -- and the survival rate of patients treated with immunotherapy using Lunit-SCOPE, its artificial intelligence-based tissue analysis system.
Korea Biomedical Review met with two Lunit executives on Tuesday to discuss the company's presentation at ASCO in detail as well as its plans and goals. They are Paeng Kyung-hyun, co-founder and head of precision pathology division, and Ock Chan-young, Lunit's medical director.
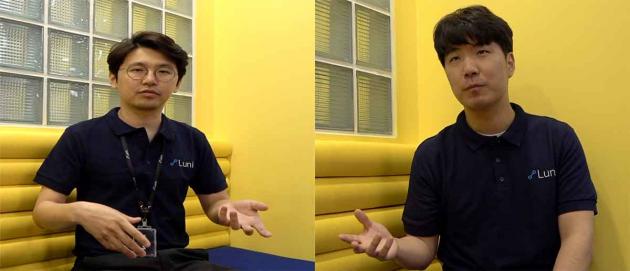
KBR: The poster showed that Lunit-SCOPE used 1,824 images provided by Samsung Medical Center in its deep learning process. Is the amount sufficient to distinguish the three types of immune phenotypes? And is there any point in the image where the classification is ambiguous?
Paeng: We are also not satisfied with the current data.
The slide area provided by the hospital is vast. If we define the slide as a whole, the sensitivity can decrease. To reduce such inaccuracies, Lunit divided the large area into smaller parts and used the results to determine the patient's immune phenotypes.
The company is working to improve its algorithm to provide better results.
Ock: The tumor microenvironment area per slide is different, so the gap is wide. Since we divided the 1,824 images into about 500 to 1,000 areas for each slide, however, we have analyzed more than 100,000 images.
The small sample was the most worrying part for the company. Under the limited sample, we believed that dividing the images into smaller sections for our deep learning process was the best way to obtain objective and meaningful results.
KBR: The poster study showed that the three classifications were optimized through the clinical results of 119 patients treated with immunotherapy. Afterward, your company tested 62 patients enrolled in the LC-biomarker study to verify the results. However, isn't the number of patients too small to verify?
Ock: At the time of ASCO 2020, the patient pool was small, as it was an interim result where we only gathered the data from Samsung Medical Center.
We are continuing to collect updated data from Samsung Medical Center while discussing with five other medical institutions – three local and two foreign ones – to receive more data.
In the end, we plan to validate our results by combining data from about six institutions. Therefore, you can expect the patient pool to increase in our final results.
Also, while the current data is a retrospective analysis of patients who have been treated at the clinical site, our final goal is to see the treatment results for the immunotherapy through prospective observational studies or tests.
KBR: When can we see the final results for the retrospective and prospective studies?
Ock: For the retrospective analysis, we plan to gather all the data and publish a peer-reviewed journal by the first half of next year. Regarding the prospective study, we will start conducting the trial from next year.
However, the trial results will take some time as we'll have to recruit patients and analyze the results.
KBR: In Korea, the use of immunotherapy is now based on the PD-L1 expression rate. For this reason, some criticize that this standard has resulted in excluding some patients who may have an effect on immunotherapy from treatment. If Lunit's classification method is applied, patients with low PD-L1 expression rates may be classified as patients who may benefit from immunotherapy. At the same time, can we assume that there will be no response to immunotherapy for patients with high PD-L1 expression rate with excluded phenotype predict?
Ock: The issues you mentioned are different when using immunotherapy as a first- or second-line treatment. This is because reimbursement based on PD-L1 expression is different.
Concerning its as second-line treatment, the reimbursement policy had been based on PD-L1 expression until recently. After specific treatments gained reimbursement as second-line treatment regardless of PD-L1 expression last year, however, there have been almost no cases of lung cancer patients who are restricted from receiving reimbursement when using immunotherapy as a second-line treatment.
However, there are still unmet needs as some patients, who are well treated with immunotherapy, are not covered by insurance when using the treatments as a first-line treatment.
In that regard, we believe the company's research is a study that provides evidence that the government should expand insurance benefits for the patients respond well to immunotherapy, regardless of PD-L1 expression.
Also, although it is prudent to exclude patients who do not show efficacy toward the treatment through this test, our study is more about classifying patients who need to use immunotherapy as monotherapy and those who need a combination treatment of immunotherapy and chemotherapy.
KBR: What is Lunit’s ultimate goal? Does your company want these three immune phenotype classifications, along with PD-L1, to be a key criterion in determining whether patients should receive immunotherapy treatment?
Ock: There is a limit to using PD-L1 to determine whether a patient is better suited to be treated immunotherapy as monotherapy or the combination of immunotherapy and chemotherapy.
Patients with PD-L1 expression of 1 to 49 percent account for 30 to 35 percent of the total. However, there are no special guidelines and reimbursements on how to treat these patients and no insurance.
Therefore, our primary goal is to solve this area and use Lunit SCOPE to provide a guideline for classification if a patient is better suited to use immunotherapy as monotherapy or the combination of immunotherapy and chemotherapy.
The second goal is to provide a guideline for patients who have their immune phenotype change after immunotherapy therapy. There are now no guidelines that suggest if the patient should stay on course or change treatment if their immune phenotype changes after treatment. We plan to provide evidence-backed suggestions toward the problem.
The company also plans to expand the range of Lunit SCOPE to other cancers.
KBR: Currently, various attempts have been made to increase the response rate of the immunotherapy, such as using other immunotherapy or chemotherapy. Shouldn't the correlation between these three phenotypes suggested by Lunit and other therapies be investigated as well?
Ock: We feel the same way. However, we don't expect it to be applied to all drugs. The platform will be a good fit for drugs with a mechanism to control immunity, especially tumor microenvironment.
Therefore, the Lunit-SCOPE three immune phenotype platform aims to go after the mechanism rather than attacking the immune-related tumor itself.
KBR: Are there any additional comments that the company would like to make to potential future partners?
Paeng: We believe that Lunit has advantages over its competitors in this field.
Rather than merely collecting and developing data, the company is also putting a lot of effort into inserting medical knowledge. It is also making technical efforts to supply and receive data efficiently, allowing our AI technology to do well on its given task.
Lunit also believes that the company is clinically validating its algorithm step by step.
To this end, the company plan to release additional research results at global conferences shortly.