KAIST said on Thursday that its researchers have optimized an AI-based drug-drug interaction (DDI) platform to predict potential adverse reactions of drugs using Paxlovid as an example.
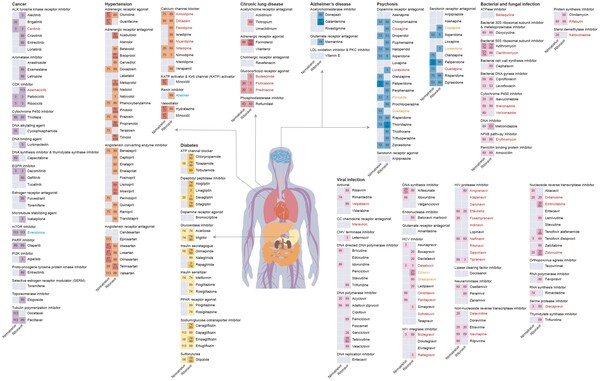
The research team, led by Professor Lee Sang-yup at the Department of Chemical and Biomolecular Engineering (BK21 four) of KAIST, improved the existing DeepDDI2 platform to predict a total of 113 drug interactions, 86 more than the previous platform, DeepDDI.
Paxlovid (ingredient: nirmatrelvir, ritonavir) is a treatment for mild-to-moderate Covid-19 patients developed by Pfizer and was granted emergency use approval (EUA) by the FDA in December 2021.
However, the FDA also issued an accompanying notice on 108 drugs that might exhibit potential drug interactions with Paxlovid and the EMA also reported 128 drugs that can potentially interact with Paxlovid.
Chronic patients suffering from high-risk hypertension and diabetes are already taking drugs, which can negatively interact with Paxlovid ingredients that have not been sufficiently analyzed for adverse drug reactions.
Accordingly, the research investigated the DDI between the ingredients of Paxlovid and previously 2,248 approved drugs using DeepDDI2. The results showed that ritonavir would interact with 1,403 approved drugs and nirmatrelvir with 673 approved drugs.
Subsequently, the team proposed alternative drugs with the same mechanism but lower drug interaction potential than approved drugs with high drug interaction potential. Accordingly, we proposed and. Some 124 alternative drugs were proposed to reduce the likelihood of drug interactions with ritonavir and another 239 alternative drugs for reducing DDIs with nirmatrelvir.
“Our platform can be used as a tool for drugs developed in emergencies such as a pandemic to help specialists anticipate potential adverse drug outcomes resulting from drug interactions and also aid drug prescription,” said Lee. “It is expected to play an important role in digital healthcare, precision medical and pharmaceutical industries by providing useful information for new drug development and prescription.”
The study was published in the Proceedings of the National Academy of Sciences journal on Mar. 13.