VUNO, a medical artificial intelligence (AI) company, said on Wednesday that a multi-center clinical research paper demonstrated the clinical efficacy of its AI medical solution, VUNO Med-DeepCARS.
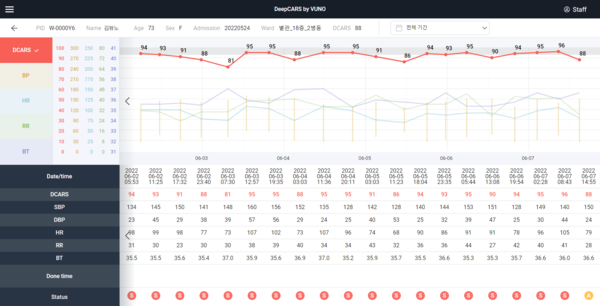
This marks the first prospective study to demonstrate the superior predictive performance of VUNO Med-DeepCARS for high-risk patients, and it is consistent with the results of previously conducted retrospective studies.
VUNO Med-DeepCARS provides a 0-100 risk score of inpatient cardiac arrest in the general wards within 24 hours through vital signs, which helps effective patient monitoring and risk management.
Prospective studies utilizing real-world data are highly trusted by medical practitioners as they are obtained from real-world clinical settings where various variables exist. Until now, most studies on AI medical devices have been retrospective, utilizing medical data from a previous point.
The VUNO research team conducted a multicenter study using data from patients admitted to the general wards of four tertiary medical institutions of different sizes, locations, and medical environments. The participating hospitals included Seoul National University Hospital, Seoul National University Bundang Hospital, Inha University Hospital, and Dong-a University Hospital.
Each hospital analyzed a total of 55,083 patients admitted to general wards over three months, comparing the performance of high-risk patient prediction systems such as national early warning score (NEWS) and VUNO Med-DeepCARS in predicting in-hospital cardiac arrest (IHCA), unplanned intensive care unit transfer (UIT) admissions, and false alarm rates.
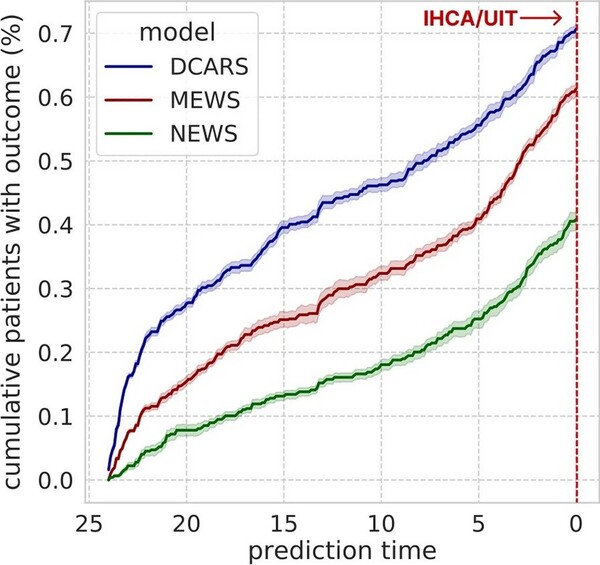
Consequently, the study found that the AUROC scores of VUNO Med-DeepCARS, a performance indicator of prediction accuracy, were superior at 0.869 compared to the existing NEWS and modified early warning score (MEWS) methods which had an AUROC of 0.767 and 0.756 respectively.
Additionally, the mean alarm counts per day per 1,000 beds were significantly reduced for the VUNO Med-DeepCARS, and the rate of appropriate alarms was higher when using the DeepCARS than when using conventional systems, demonstrating the high reliability of the alarms.
The study also found that VUNO Med-DeepCARS was effective without any limitations on patient age, gender, or time of occurrence.
The study provides further evidence that VUNO Med-DeepCARS, which is currently being rapidly deployed in healthcare, can dramatically improve inpatient safety management in real-world settings, said a VUNO official.
"We will continue to build clinical confidence in the product by conducting additional prospective studies, and will do our best to ensure that it is adopted in more healthcare settings as an essential tool to protect patient safety," said VUNO CEO Lee Ye-ha.
The study was published in the Critical Care journal of BioMed Central (BMC) on Sept. 5.
Related articles
- VUNO’s cardiac arrest-predicting device now available for children under 19
- AI-powered cardiac arrest prediction system saved this patient's life.
- VUNO founder launches AI eye disease management startup
- FDA OKs VUNO's brain imaging analysis device
- VUNO to disclose study results of AI-based ECG analysis software at AHA 2023
- VUNO’s Q3 sales surge with success of AI-based cardiac arrest management system