Korean doctors have developed a model to predict postoperative scar severity using artificial intelligence (AI).
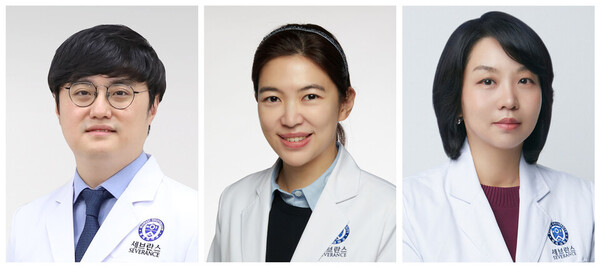
A research team -- led by Professors Kim Je-min and Kim Ji-hee of the Department of Dermatology at Yongin Severance Hospital and Professor Lee Ju-hee of the Department of Dermatology at Severance Hospital -- said Thursday that they have developed a post-surgery scar severity prediction model using deep learning-based image analysis.
The research team conducted a study to create a deep learning integrated model based on image and clinical data that can more accurately predict scar severity.
The study used data from 1,283 patients who visited the Department of Dermatology at Severance Hospital and Yonsei Cancer Hospital's Scar Sculpting Laser Center for scars after thyroidectomy. Scar severity was classified based on the Vancouver Scar Score (VSS) and the clinical judgment of three dermatologists specializing in scars.
The researchers classified data from 1,043 patients who visited Yonsei Cancer Hospital's Scar Sculpting Laser Center for scars left after thyroidectomy between December 2015 and December 2021 as the main dataset, and data from 240 patients who visited Severance Hospital's Dermatology Department for scars left after thyroidectomy between December 2010 and July 2015 as the external test set.
The AI model was validated on the internal and external test sets, which were segmented from the main dataset, and 16 dermatologists were presented with images of scars from the internal test set, assessed for severity, and compared to the performance of the AI model.
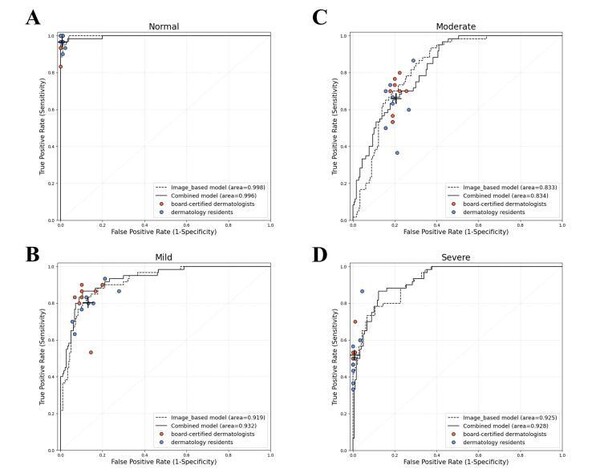
The results showed that the area under the receiver operating characteristic curve (ROC) for the image-based model using the internal test set was 0.931, which increased slightly to 0.938 when combined with clinical data. The area under the ROC for the combined image and clinical data prediction model using the external test set was 0.896 and 0.912, respectively.
The performance of the model evaluated based on the internal test set images was similar to that of the 16 dermatologists. This suggests that AI models derived from images and clinical data can effectively predict postoperative scar severity.
"The AI model developed in this study will significantly improve the accuracy of scar diagnosis, helping to make objective and reliable decisions in the clinical setting," Professor Kim Je-min said. "We look forward to improving patient treatment methods and creating more predictable treatment outcomes."
The study was recently published in Nature's sister journal, Scientific Reports.
The team believes that the high-quality, large-scale, standardized post-surgical scar dataset and severity assessment algorithm developed in this study could be extended to assess the severity of other dermatofibrosis diseases and predict treatment response, and is pushing to develop additional models.