VUNO, a Korean medical AI company, said Wednesday that its research on a self-supervised learning (SSL) algorithm to improve the efficiency and accuracy of artificial intelligence-based ECG data analysis has been adopted by the International Conference on Learning Representations (ICLR).
ICLR, now in its twelfth year, is an annual conference dedicated to sharing the latest advances in artificial intelligence technology and research, and the most prestigious international society in deep learning.
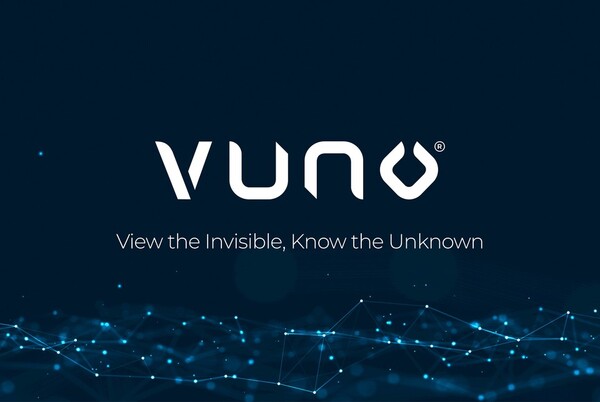
The VUNO team proposed a self-supervised deep learning model specialized for learning and analyzing electrocardiogram data in the study. Self-supervised learning is a deep learning technology that is the basis of super-scale AI, such as ChatGPT, meaning that AI finds rules by itself and analyzes unlabeled data to present results. Labeling refers to processing data into a learnable form to create a kind of answer sheet when developing an AI model.
The researchers wanted to see if self-supervised learning could reduce the costly labeling process in healthcare and provide accurate results with a small amount of data.
They first built a self-supervised learning model trained on many unlabeled ECG data. They tested whether the model could effectively identify several cardiovascular diseases, including arrhythmias and myocardial infarction when analyzing ECG labeling data measured differently.
Based on AUROC (Area Under ROC Curve), a performance measure of prediction accuracy, the model performed well on standard 12-lead ECGs measured in hospitals, with an AUROC of 0.933, 0.903 on six-lead ECGs, and 0.804 on single-lead ECGs.
It also performed well with a small amount of data. Based on 12-lead ECGs, it achieved an AUROC of 0.878 using only 5 percent of the training data of a typical deep-learning model. When trained on 1 percent of the labeled data (about 100 labels), VUNO's model achieved an AUROC of 0.815, significantly more accurate than a typical deep learning model (AUROC of 0.615).
"These results show that our self-supervised learning model has the versatility to detect a wide range of cardiovascular diseases with only a small amount of data, regardless of how the ECG is measured," VUNO said. "It can significantly reduce the labeling process when developing artificial intelligence models, which can contribute to reducing R&D costs."
VUNO plans to apply the model to its electrocardiogram business in the future. It will be applied to commercializing disease-specific detailed models of VUNO Med-Deep ECG, an AI-based ECG analysis software currently undergoing domestic confirmatory clinical trials.
"The research adopted by the ICLR is of great significance not only because it reflects the latest artificial intelligence trends in the field of electrocardiography, but also because it demonstrates results that can be of practical help to our future business," VUNO CEO Lee Yeha said. "Electrocardiography is an area with high potential as a clue to various diseases, and we will continue to conduct research and development to become a company that can provide high-quality healthcare services anytime, anywhere."
Related articles
- VUNO marks record revenue, eyes break-even point with rapid growth in cardiac arrest prediction
- VUNO's AI cardiac arrest prediction solution sets monthly record for new contracts, bed allocations
- VUNO to participate in Arab Health 2024
- VUNO's AI chest CT reading solution wins reimbursement in Japan
- [RSNA 2023] VUNO will break even in the third quarter of 2024: CEO
- VUNO partners with DRGEM to enhance X-ray diagnostics worldwide
- Korean medical device makers shine at Europe's largest radiology conference
- VUNO registers US trademark for VUNO Med-DeepCARS
- VUNO introduces cardiac arrest risk monitoring device at critical care society’s meet
- VUNO's AI-based ophthalmic diagnostic SW receives key regulatory approval