Researchers at Severance Hospital have developed a machine learning-based fractional flow reserve (FFR) prediction technology using optical coherence tomography (OCT) images and patient biometric information.
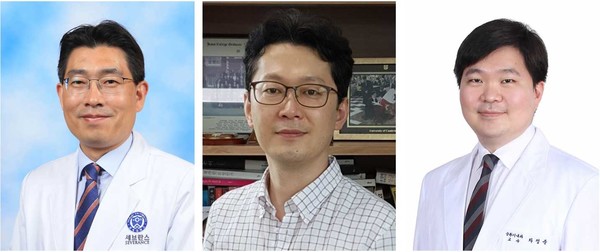
The hospital expects that the new technology will help treat patients receiving coronary intervention surgery.
Coronary artery disease has two types’ angina and myocardial infarction. Angina is an illness in which pain occurs due to insufficient blood supply to the heart due to narrowing of the coronary artery, myocardial infarction is an illness where the heart muscle is completely blocked.
Its treatments include medication, intervention, and coronary artery bypass surgery. Among treatment methods, hospitals widely use double coronary intervention, medically treating blocked or narrowed cardiovascular vessels by piercing a blocked coronary artery. In patients with intermediate stenosis, hospitals perform stent implantation after determining the stenosis of blood vessels.
For accurate stent insertion, patients usually undergo an OCT test, an imaging method capable of imaging microstructures inside the vascular tissue, and the FFR test, which provides intravascular blood pressure information.
To speed up the process, the team, led by Professor Kim Jung-sun at the hospital, developed a diagnostic technology that can predict the FFR value using machine learning for the vascular stenosis information and the patient's biometric information using the OCT diagnostic test.
Professors Cha Jung-joon at Korea University Anam Hospital and Ha Jin-yong of the department of Sejong University also participated in the study.
In developing the software, the team sampled 125 patients undergoing coronary intervention at Severance Hospital from November 2013 to January 2015 and measured OCT and FFR values in four groups. Afterward, the team used the data on 104 patients for its machine learning system.
Among them, the FFR value of 21 patients was predicted through six important characteristics --the coronary artery inner diameter at the narrowest area, the ratio of the stenosis site, the length of the lesion, the coronary artery inner diameter near the body, the number of platelets before the procedure, and hypertension.
As a result of comparing the FFR value obtained through machine learning with the actual measured FFR value, the team found the data similar to the real measured value.
"The results of this study are expected to reduce the burden and side effects of patients undergoing coronary intervention," the team said. "Notably, the FFR value can be predicted within one to two minutes through computer simulation instead of the conventional invasive FFR test, which is expected to improve diagnosis and treatment results for patients with coronary artery disease who struggle with time."
The research team stressed that they plan to develop the FFR simulation software and launch it in the cardiovascular disease diagnostic medical imaging software market, which has grown rapidly.
"Thanks to this study, the process of having to separately insert a pressure wire into the coronary artery to measure FFR can be quickly checked through machine learning in the clinical field," Professor Kim said. "This study has shown potential, and the use of clinical data from more patients in the future will lead to better clinical results."
Scientific Reports published the result of the study.